Stylization
TextBoost also offers high-quality personalization of style!
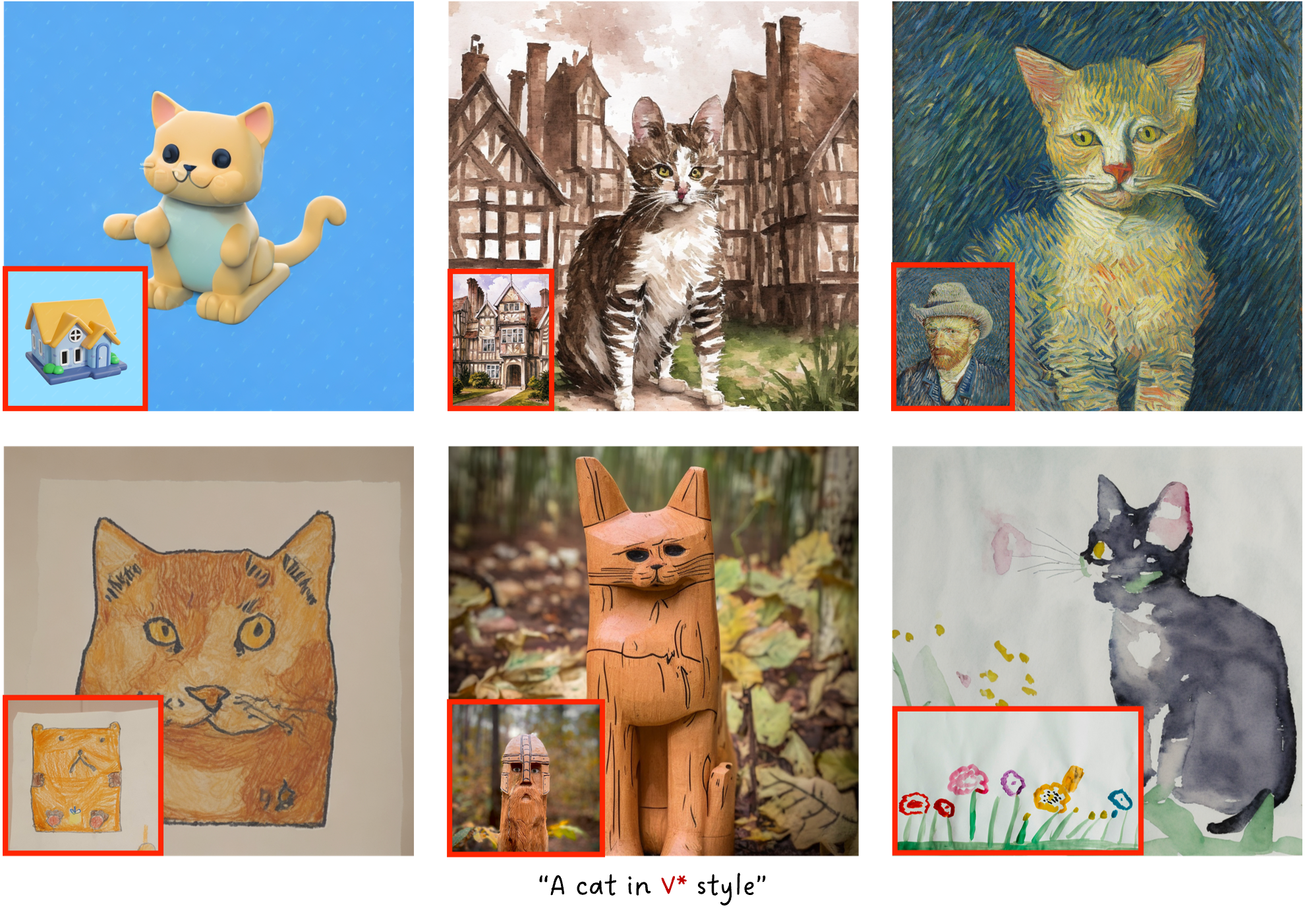
Recent breakthroughs in text-to-image models have opened up promising research avenues in personalized image generation, enabling users to create diverse images of a specific subject using natural language prompts. However, existing methods often suffer from performance degradation when given only a single reference image. They tend to overfit the input, producing highly similar outputs regardless of the text prompt. This paper addresses the challenge of one-shot personalization by mitigating overfitting, enabling the creation of controllable images through text prompts. Specifically, we propose a selective fine-tuning strategy that focuses on the text encoder. Furthermore, we introduce three key techniques to enhance personalization performance: (1) augmentation tokens to encourage feature disentanglement and alleviate overfitting, (2) a knowledge-preservation loss to reduce language drift and promote generalizability across diverse prompts, and (3) SNR-weighted sampling for efficient training. Extensive experiments demonstrate that our approach efficiently generates high-quality, diverse images using only a single reference image while significantly reducing memory and storage requirements.
With the same text prompt, TextBoost generates a variety of images featuring the subject.
TextBoost also offers high-quality personalization of style!
@article{park2024textboost,
author = {Park, NaHyeon and Kim, Kunhee and Shim, Hyunjung},
title = {TextBoost: Towards One-Shot Personalization of Text-to-Image Models via Fine-tuning Text Encoder},
journal = {arXiv preprint arXiv:2409.08248},
year = {2024},
}